D-Shaing GoJ Project ❶Daytime Shift Nudge for Households
D-Shaing GoJ Project ❶Daytime Shift Nudge for Households
Following new location standards, D-Sharing demonstrated a strong evidence to promote consumers' timeshift by evaluating CO2 reduction value.
Objectives of the Government Funded Nudge Project
To use a model that has been put into practical use in industrial applications in anticipation of regulatory updates due to the revision of the 2025 GHG Protocol Scope 2 Guidance
In the industrial sector, we have pioneered the commercialization of our technology, which manages and evaluates each consumer's CO2 emission reduction behavior in terms of reduction in electricity consumption (kWh) and reduction in period/weighted average consumer CO2 emission factor (kG-CO2/kWh), on a volunteer basis for pioneering companies worldwide.
On the other hand, in the residential sector in Japan, its commercialization and implementation in society must await the revision of laws and regulations. This is because, unlike industrial applications, the number of consumers who are willing to incur additional expenses in advance of the legal requirements is limited. However, since the GHG Protocol Scope 2 Guidance will be drafted and released in 2024 and finalized as early as 2025, the Ministry of the Environment is considering commercialization in 2026, and is building a nudge model under the Ministry of the Environment's Nudge Demonstration Project, which is based on the assumption that the regulations will be revised. The Ministry of the Environment is currently building a nudge model under the Ministry's Nudge Demonstration Project based on the assumption that the regulations will be revised.
The Ministry of the Environment is currently working on the construction of a nudge model under the Ministry's Nudge Demonstration Project, which is premised on the revision of the relevant laws and regulations.
The purpose of the "Energy Conservation Nudge at Home" experiment is to collect digitally and objectively information on actual household energy use and the status of energy conservation behavior, etc., and to conduct sophisticated analysis of CO2 emission reduction effects through the use of AI, etc., so that the public can visualize the usage history and future usage forecasts for each electricity load, and to use the knowledge of behavioral science such as nudges in response to their behavior. The objective is to construct a BI-Tech model that promotes advanced behavioral change toward decarbonization by proposing comfortable and eco-friendly lifestyles suited to each individual, such as by providing monetary and non-monetary incentives based on the knowledge of behavioral science such as nudges, and to demonstrate the model using randomized controlled trials (RCT) methods. The aim is to demonstrate the model through randomized controlled trials (RCTs) and other methods.
In FY2022, we conducted a preliminary demonstration experiment on the effects of monetary and non-monetary incentives on electricity-saving behavior, and found that monetary incentives led to an additional 4.3% reduction in electricity consumption and CO2 emissions. The results showed a statistically significant 4.3% additional effect of monetary incentives on electricity saving and CO2 emissions reduction. The following is a summary of the results.
Period of the experiment
November to December 2022
Participating households in the experiment and intervention details
Approximately 700 households living within the service area of Chubu Electric Power Company were randomly assigned to one of the following three groups.
During the implementation period of the demonstration experiment, each household was asked to conserve electricity by presenting its forecasted daily electricity consumption and estimated electricity consumption for each electrical appliance and other electrical equipment, and to report on its daily energy-saving behavior, with a ranking based on the number of energy-saving actions taken. Group 1: A group in which a ranking is displayed (intervention group 1)
In addition to the intervention content of Intervention Group 1, a group that provides financial incentives when actual daily electricity consumption falls below the estimated electricity consumption (Intervention Group 2)
A group that does not provide nudges as a comparison (control group)
■ Overview of the AI used
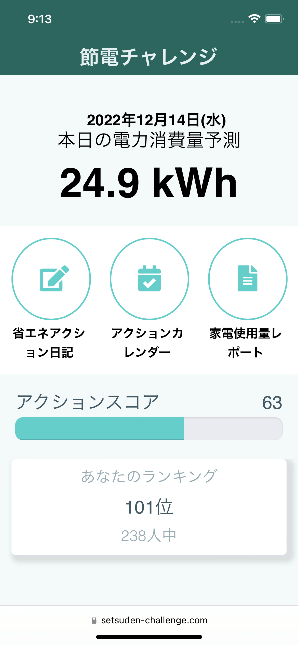
Prediction of electricity consumption based on electricity consumption and weather data for the past two years, current weather forecasts, and attribute information for each household (Figure 1)
The estimated power consumption of each electrical device such as home appliances is analyzed using machine learning and device separation technology (Figure 2).
Figure 1: Example of electricity consumption forecast
https://www.env.go.jp/content/000145287.png
Figure 2: Example of estimated power consumption for each electrical device
https://www.env.go.jp/content/000145288.png
Results
In a comparison between intervention group 1 and intervention group 2, a statistically significant 4.3% additional electricity and CO2 savings was demonstrated by the addition of financial incentives. On the other hand, a comparison between the control group and intervention group 2 showed a trend toward a 2.7% decrease in electricity use with the intervention, but no statistically significant difference was detected.
Path toward future social implementation
In the future, MHI intends to consider the following as its policy to establish a path toward social implementation
Ahead of the revision of the GHG Protocol Scope 2 Guidance and its implementation in Japanese regulations, we will collect and analyze big data focusing on the differences in CO2 emission coefficients of power transmission and distribution networks associated with power consumption in each time period and power transmission and distribution network to reduce electricity prices, ensure supply and demand balance, and reduce CO2 emissions of the entire power system in an integrated manner. The model will demonstrate the effectiveness of promoting energy conservation and time-shifting in order to reduce electricity prices, ensure a balance between supply and demand, and reduce overall grid CO2 emissions in an integrated manner.
In doing so, we will analyze household attributes and our technology's period/weighted average consumer CO2 emission coefficient (kG-CO2/kWh) to categorize which households with which attributes and attitudes emit more CO2, and build a nudge intervention model for each type.
Then, the independently developed system will be improved to visualize the expected future consumption, CO2 emissions, electricity rates (baseline), etc. for each household using AI, and have the households work on energy conservation and time-shifting so that they will fall below the baseline. Demonstrations to clarify which intervention methods are effective will be conducted through RCTs and other means.
In addition, price elasticity of electricity consumption and reward sensitivity will be elaborately analyzed for each consumer attribute and awareness, and the effectiveness of intervention measures to improve sensitivity, including point services, will be verified. In addition, a new intervention model, such as a game against foreign consumers, will be developed and its effectiveness will be preliminarily demonstrated.
DR Nudge: Build an intervention model for the commercialization of virtual power plants with up and down demand response that contributes to decarbonization, and demonstrate its effectiveness through multiple RCTs and other measures.